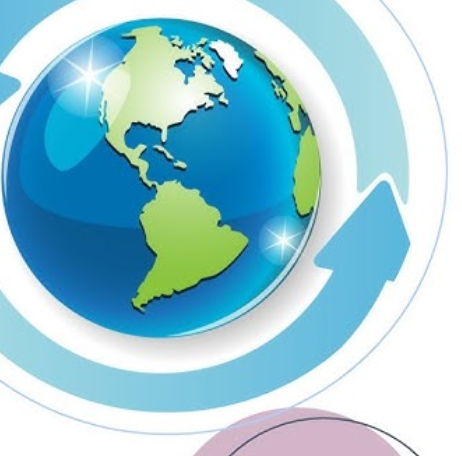
This course provides an understanding of the role of environmental cost accounting in modern businesses.
Learning outcomes: The student is aware of the importance of environmental cost accounting in modern businesses.
The student develops basic knowledge of the types of ecological costs related to the use of the environment.
The student is able to apply tools of environmental cost accounting in modern ecological projects.
- Teacher: Anna-Maria Kaminska
- Teacher: Tatiana Pasko
- Teacher: Politechnika Wroclawska
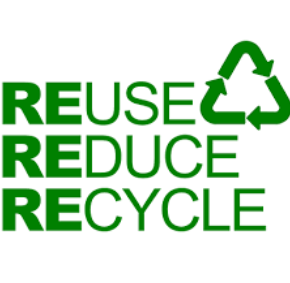
Course purpose: introduce students with the global environmental issues caused by household waste in the world; introduce to students sustainable development goals related to waste management; introduce students with household waste categories and labeling; develop students' ability to sort household waste by category; develop in students a sense of awareness that sorting waste can improve the global situation; provide students with the understanding how to recycle the waste at home (upcycling) and reuse it; develop students' sense of responsibility and environmental awareness in order to ensure the principles of sustainable development.
- Teacher: Anna-Maria Kaminska
- Teacher: Khmelnytskyi National University
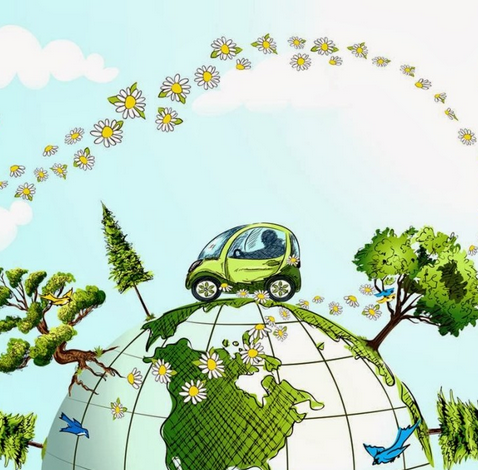
Ecotourism as a tool to help in environmental protection, local community building, economic growth, and sustainable development.
Purpose of the course:
Participants could find and process information about sustainable tourism practices and their impact on the natural environment, as well as see the role and need of local communities for the socio-economic and cultural development of the region.
Course description:
Sustainable tourism can be considered as one of the active fields that address many social, cultural, economic and environmental issues and try to harmonize them. During the course, students will learn how environmentally friendly practices and responsible forms of travel can contribute to environmental sustainability and improve the quality of life in society. Participants will learn about the types of eco-certification, the role of local communities and initiatives to create a healthy tourism product. One of the directions of the course will concern urban development, innovative tourism infrastructure and strategies to deal with the consequences of climate change. The basic principles of sustainable development of the United Nations became one of the inspirations to promote and put these principles into practice within the framework of this course. Through a mix of theoretical knowledge and practical examples in this course, students will learn about the international definitions of ecotourism in protected areas and their relevance to local realities. In the course, special attention will be given to successful global and local projects that are distinguished by regenerative and holistic approaches, including the concept of ecovillages, the global ecovillage network, the first ecovillage in the Caucasus region, which is considered one of the most important trends in achieving the UN's sustainable development goals. Different studies, articles, textbooks were used in the preparation of course topics. Also, the situational analysis of the ongoing environmental protests, where the tourist infrastructure is planned against the will of the locals.
At the end of this course, participants will be able to:
To see the importance of involvement of local communities in sustainable tourism.
Situational analysis of innovative tourist infrastructure.
Identify adaptation and mitigation strategies for tourism as a result of climate change.
To re-evaluate the cultural and traditional dimensions of tourism in the prism of sustainability.
Finding contacts for global cooperation and reproducing successful examples of sustainable tourism projects.
To learn about global ecovillage network projects and get involved in the local ecovillage development process
The need for local production, waste management and recycling in tourism products.
Applying the principles of sustainable horticulture, permaculture and regenerative agriculture in the creation of tourism services.
To distinguish and explore nomadic forms of tourism.
Develop strategies for sustainable tourism management to balance issues such as gentrification, drinking water scarcity and urban runoff.
To create sustainable tourism products that comply with the principles of sustainability and meet the needs of the modern traveler.
This course is intended for tourism practitioners, enthusiasts, environmentalists, urban planners, those interested in green policy and anyone interested in promoting sustainable travel practices and wants to contribute to the protection of the environment.
- Teacher: Anna-Maria Kaminska
- Teacher: Giorgi Tchotchua